Genome-wide association studies, also known as GWAS, have been used extensively over the last 5 years by genetic researchers.
GWAS are generally used to allow researchers to discover variants in genomes that indicate the presence of a particular disease.
With this information, researchers can begin to understand how genes contribute to diseases and they can begin to develop therapies and treatments.
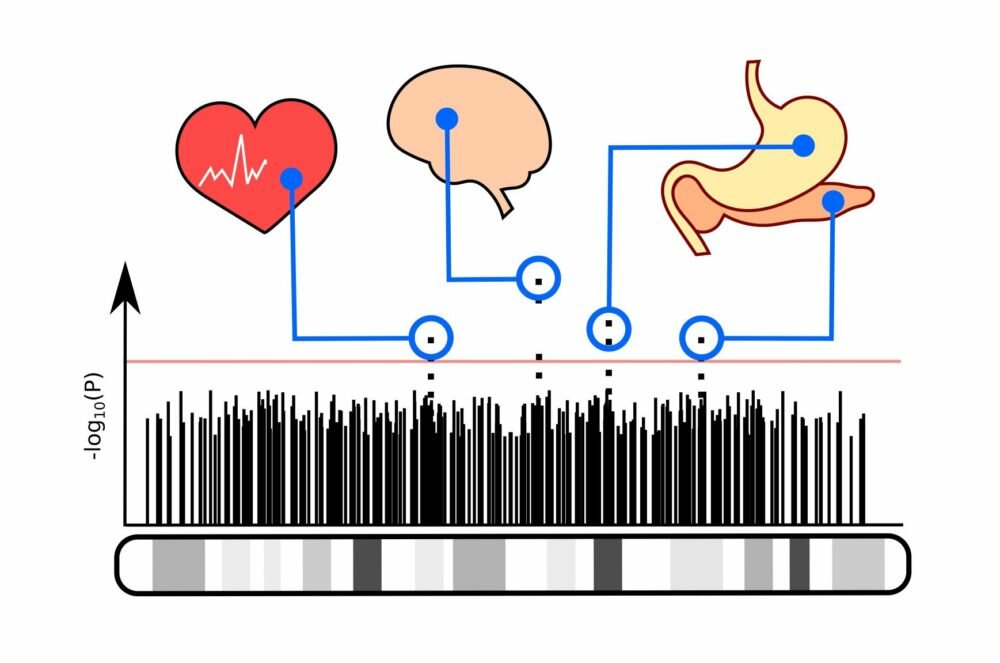
Reading these studies can be difficult but relying on mainstream media reporting can be misleading.
To help you out, we’ve put together a bit of a crib sheet that will help you access and assess the information in these studies.
There are a number of key things you need to focus on to establish whether a study is worth the paper it’s written on.
Key Things
1. Sample size
The sample size of a study is very important in general, but especially with GWAS. This is because GWAS are designed to establish very small variants.
They need a huge range of samples to be able to say with some confidence that small differences have any statistical significance.
Papers with fewer than 1000 cases and control samples should raise your suspicions. Studies in other areas can, in some cases, use smaller sample sizes but GWAS need larger samples.
2. Quality control
One of the difficulties with GWAS is being able to collect clean and relevant genotype data. You want to make sure that the standard quality control metrics have been observed.
These should include genotype call rate, Hardy-Weinberg equilibrium.
You also want to check whether they have focused their quality control efforts on the genotypes most associated with SNPs.
Despite there being years of practice and research into lab problems that may affect data, some researchers have missed QC issues.
3. Confounders
Confounding factors are things that may give false results. They are variables in a study that are different between samples and controls.
They generally are not responsible for creating the disease but may, accidentally, be attributed as a factor that influences the disease.
Consider the following scenario, a disease is prevalent in one country but less so in another country. Researchers use GWAS to try and establish what causes this disease.
The different populations of each place have different genetic markers as they have different genetic ancestry.
Researchers may presume that the different genetic markers are an indication of the disease but this is actually a confounding factor.
The disease may be more prevalent in one place because of poor hygiene practices or over population.
Other confounding factors might present if the cases were carried out in different laboratories or if a different collection method was used.
Commonly, a statistical tool called the ‘QQ plot’ is used to show that confounders aren’t affecting the results. For more information about QQ plots, take a look at this paper.
4. Replication
GWAS must be replicable in order to prove that they are not affected by false results or confounders.
The study must be able to be replicated by independent researchers, at independent laboratories with different samples.
It means that the technology and methodology must be able to be recreated by people who were not originally involved in the study.
5. Biology
There are some firm results generated by GWAS but there is always some sort of speculation about why the identified genes are important to the disease.
You will need to read the biological results with a critical eye because speculation can be subjective. You can unearth a paper that supports pretty much any hypothesis.