Over the course of the past year or so, I’ve been working (with Jonathan Pritchard) on a statistical method for learning about the history of a set of populations from genetic data. Much of this work is described in a paper we recently made available as a preprint [1]. However, as many readers will know, writing a paper involves deciding which results are important to the main point (and worth fleshing out in detail), and which aren’t. In this post, I’m going to describe some results and thoughts that didn’t quite make the cut, but which I think merit a small note. In particular, I’m going to discuss how having a demographic model for a large number of populations might be used to identify genes important in adaptation, and describe results from humans and dogs.
Background
Imagine you have genome-wide genetic data (from SNP arrays, genome sequencing, or whatever) from a number of populations in a species. A common way to visualize the relationship between your populations is to use a tree. For example, below I’ve built a tree of the 53 human populations from the Human Genome Diversity Panel (using the data from Li et al. [2]).
Of course, populations within a species don’t just split, they also mix via gene flow. These types of events are not modeled when forcing populations into a tree. Below, I’m showing a heatmap that depicts how well each pair of human populations fits the above tree. The dark greens, blues, and blacks represent pairs of populations that are, in some sense, too far away from each other in the tree. These populations are potential candidates for admixture events (indeed, you can see known admixed populations like the Mozabite jump out from a plot like this). This is the sort of signal we focus on in our paper.
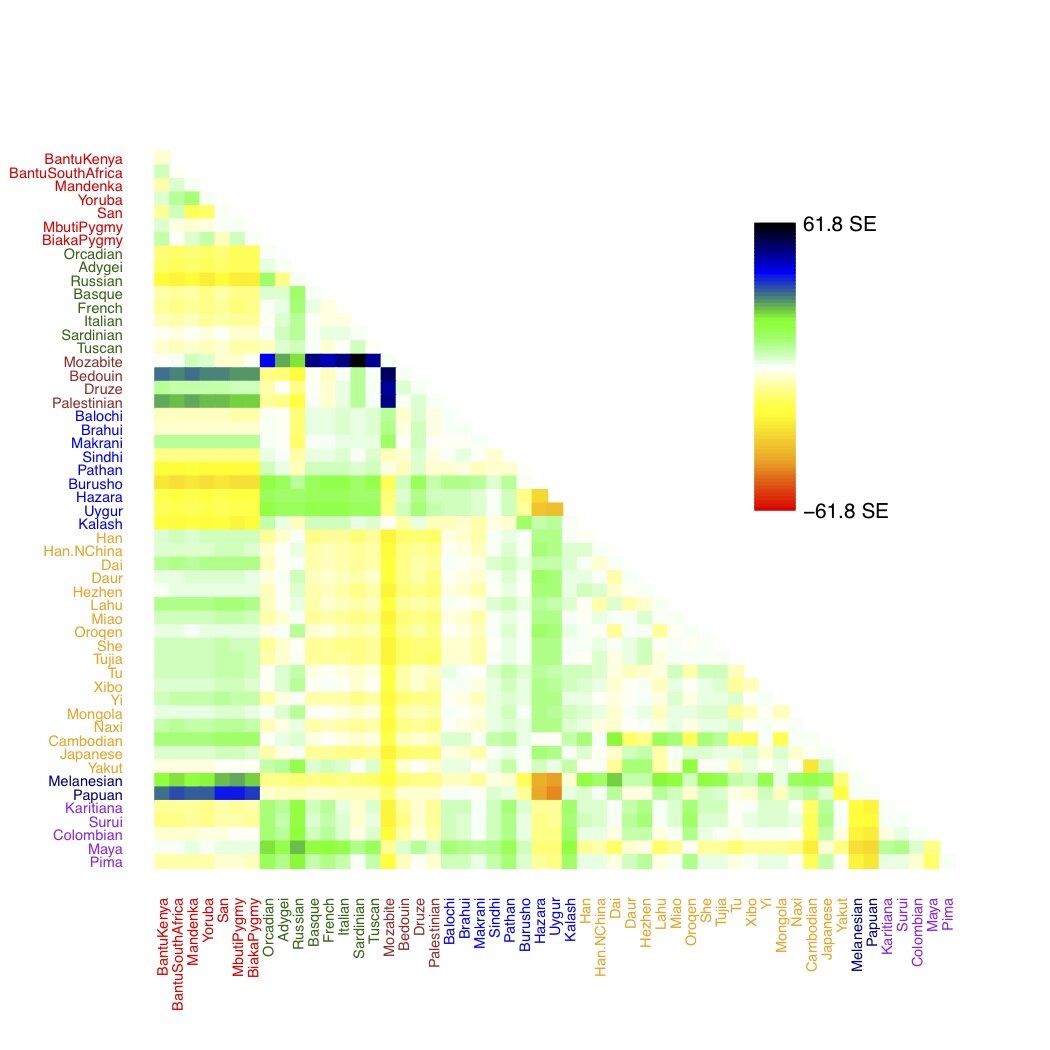
Residual fit from the tree of 53 human populations. Large residuals indicate potential admixture events.
While populations that don’t fit a tree well are candidates for gene flow, what about individual SNPs that don’t fit the tree? These SNPs are ones that have changed frequency in ways that are surprising given the demographic history of the populations. A plausible hypothesis, then, is that they (or linked variation) have been the target of natural selection.
Results
To explore this possibility, I used the human data from Li et al. [2] and dog data (from 82 dog breeds) from vonHoldt et al. [3]. I first built trees of the populations in each species. The human tree is the one shown above, and the dog tree is the one from our paper. I then applied a simple metric that measures how well the allele frequencies at any given SNP match the tree [4]. The “interesting” SNPs are those with the worst fit to the tree. Below, I’m showing the 10 most “interesting” SNPs from the dog data; I report their chromosomal position, the nearest gene, and the phenotype influenced by variation in this region (if one is known). I made no attempt to group together SNPs that tag the same signal.
Chr | Pos | Nearest gene | Phenotype |
10 | 11000273 | MSRB3 | body size |
15 | 44267010 | IGF1 | body size |
15 | 44226658 | IGF1 | body size |
24 | 26359292 | ASIP | coat color |
10 | 11017207 | MSRB3 | body size |
20 | 24889546 | MITF | coat color |
13 | 11659791 | RSPO2 | coat length/texture |
24 | 26370498 | ASIP | coat color |
13 | 11660193 | RSPO2 | coat length/texture |
1 | 96150819 | CDC37L1/AK1 | snout length |
The massive selection pressures imposed on dogs by human breeders are apparent from this analysis. Like a similar analysis by Boyko et al. [5], we observe that the most outlying SNPs are already known to influence things like body size and shape and coat color.
Now let’s look at the top 10 SNPs from the human data (links on each SNP go to maps showing their worldwide distribution):
SNP | Chr | Pos | Nearest gene | Phenotype |
rs1834640 | 15 | 46179457 | SLC24A5 | skin pigmentation |
rs260690 | 2 | 108946170 | EDAR | hair morphology |
rs10882168 | 10 | 94919424 | CYP26A1/FER1L3 | ? |
rs4918664 | 10 | 94911055 | CYP26A1/FER1L3 | ? |
rs2250072 | 15 | 46172199 | SLC24A5 | skin pigmentation |
rs6583859 | 10 | 94883463 | CYP26A1/FER1L3 | ? |
rs2384319 | 2 | 26059759 | KIF3C | ? |
rs6500380 | 16 | 46933278 | LONP2 | ? |
rs4497887 | 2 | 125576247 | CNTNAP5 | ? |
rs9809818 | 3 | 71563256 | FOXP1 | ? |
In humans, it appears much less is known about the selective pressures (assuming these outlier SNPs have indeed experienced selection). We see two of the well-established selected genes (SLC24A5 and EDAR) at the top of the list, but the remainder have no known phenotype (though I assume many of these have shown up in other scans for selection). It is plausible that these genes play important roles in the phenotypic differences between human populations.
Conclusions
An approach like that described above seems potentially promising for quickly identifying SNPs that show extreme differences in allele frequency (and thus have potentially been the targets of natural selection) in a large set of populations. This approach is somewhat more model-based than Fst, and somewhat less model-based than Bayesenv [6], and thus may be useful in some settings.
—
[1] Pickrell and Pritchard (2012) Inference of population splits and mixtures from genome-wide allele frequency data. hdl:10101/npre.2012.6956.1
[2] Li et al. (2008) Worldwide Human Relationships Inferred from Genome-Wide Patterns of Variation. DOI: 10.1126/science.1153717
[3] vonHoldt et al. (2010) Genome-wide SNP and haplotype analyses reveal a rich history underlying dog domestication. doi:10.1038/nature08837.
[4] The tree predicts a variance/covariance matrix of allele frequencies (this is W in the notation of [1]). For any given SNP, I compute the sample variance/covariance matrix (let’s call this V), and then compute the sum of squared differences between the entries of V and W. I then find the scaling factor that minimizes this sum of squares; i.e., I find the scalar x that minimizes the sum of squared differences between the entries of V and xW. The remaining sum of squared differences is a measure of the “badness of fit” of the SNP to the tree. Obviously there are a number of complications to the interpretation of this number (e.g., it will be larger for SNPs with a larger x, and I make no attempt at accounting for the correlation between different entries of the matrix).
[5] Boyko et al. (2010) A Simple Genetic Architecture Underlies Morphological Variation in Dogs. doi:10.1371/journal.pbio.1000451.
[6] Coop et al. (2010) Using Environmental Correlations to Identify Loci Underlying Local Adaptation. doi: 10.1534/genetics.110.114819.
Hi Joe. Nice post and congrats on getting the paper out.
We experimented with something similar when we developed Bayenv. We first estimated the neutral covariance of allele frequencies using genome-wide SNPs. At each SNP we then MCMC’d over population allele frequencies and computed the likelihood under our null model. This likelihood was then compared to that under a model where the population allele frequencies were set to their MLEs given the sample and the sample was just binomial drawn from those frequencies. This gave us a LR to use as a goodness of fit statistic. This statistic allowed us to spot loci that were poorly fit by the neutral covariance model. when applied to the HGDP data it pulled out the usual suspects including KITLG and SLC24A5. Like you, we decided against including this in the main paper and focused on environmental correlates. In the end I felt like the method provided a good alternative to global Fst for spotting interesting allele frequencies but was very hard to interpret why a SNP was an outlier. Putting it on a tree may well work better for providing a explanation of which lineages appear to have “drifted” too much at particular SNPs.
Interesting. FOXP1 is contained one of the regions they pulled out in the Neandertal genome paper as being under selection.
I’m with Nick, the FOXP1 association stood out to me at first glance. It’s been associated with language development and mental retardation in human studies (e.g. Hum Mutat. 2010 Nov;31(11):E1851-60, Am J Hum Genet. 2010 Nov 12;87(5):671-8.) and also comes up in numerous studies looking at cancer incidence or prognosis.
Interesting to wonder what those other genes with ?’s are doing – the CYP gene no doubt playing a role in liver metabolism of something. The others?
Very interesting work.
Thanks for making the software and the paper immediately available to the public.
Very interesting.
Any thoughts on why the Neanderthals and Denisovans don’t show any relationship to us? All selected out but for a few SNPs?
Would it be possible to rewrite this software to create the same maps, chromosome by chromosome?
Great information on dogs. The early dogs follow a Mongolia-Central Asia-Europe trajectory, somewhat emulating Early Eurasians, although the direction is not clear.
In answering my own question about why the software currently does not pick up the contribution of Neanderthals to all non-African populations, I see that the algorithm currently relies on the assumption that the history of the species is largely tree-like. (Page 20.)
It’s very interesting that in Figure 8 in the Supplementary material, it looks like TreeMix is attempting to find the Denisovan and Neanderthal admixture events in the genetic past of Oceanic populations. Impressive, even if the result is not robust.
I can think of one population missing in this analysis: that of the inferred population implied in the paper “Genetic evidence for archaic admixture in Africa”, Hammer et al, PNAS, 2011.
Regarding Orcadian-Native American admixture: here’s the open access lecture given by Dennis Stanford on his Across Atlantic Ice hypothesis:
https://gustavus.edu/events/nobelconference/2008/stanford-lecture.php
Marnie,
Thanks for the comments. As you’ve found, there is indeed some discussion of Neandertal and Denisova mixture deep in the supplementary material :)
There is really a lot of evidence for mixture between diverged human populations in these data, so the analysis in the paper is just scraping the surface. It’s plausible TreeMix would pick up archaic admixture into Africa, but that will require a focused look at African populations, which I have not done.
Would it be possible to rewrite this software to create the same maps, chromosome by chromosome?
All TreeMix takes as input is a list of allele counts, so you could give it only those from a single chromosome. Though of course that reduces the amount of data considerably.
Chris,
I’ve driven myself insane looking at lists of genes like this in the past, so beware! :)
Graham and Nick,
I know we’ve talked offline, but again thanks for the info.
Could also sexual selection (in addition to natural selection) explain some SNPs not fitting the tree?
“While populations that don’t fit a tree well are candidates for gene flow, what about individual SNPs that don’t fit the tree? These SNPs are ones that have changed frequency in ways that are surprising given the demographic history of the populations.”
The corollary of this question would be to ask which SNPs known to underlie disease susceptibility *do* fit the tree. I’m looking at this tree and thinking that across this map, humans demonstrate genetic characteristics such as substance abuse vulnerability or susceptibility to obesity, for example. Such characteristics pose the greatest social cost as they impact the breadth of the human population. If some of these disease related SNPs are found *not* to be under selection, they might be good candidates for population aspecific drug development.
I don’t know if you know this paper, if not I think it would be worth checking it out (disclaimer: I am one of the authors :)
Bonhomme et al. 2010 Genetics Detecting selection in populationtrees: the Lewontin and Krakauer test extended
The approach to build the tree is much less advanced than treemix though.
I hadn’t seen that paper, but it definitely looks relevant. Thanks!
this info was crap and not helpful!!!